
如何在caffe中添加新的Layer
1个回答
2017-04-07 · 百度知道合伙人官方认证企业
1【专注:Python+人工智能|Java大数据|HTML5培训】 2【免费提供名师直播课堂、公开课及视频教程】 3【地址:北京市昌平区三旗百汇物美大卖场2层,微信公众号:yuzhitc】
向TA提问
关注
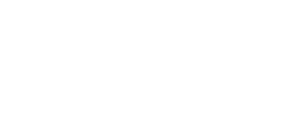
展开全部
在caffe中添加新的Layer:
Here's roughly the process I follow.
Add a class declaration for your layer to the appropriate one of common_layers.hpp, data_layers.hpp,loss_layers.hpp, neuron_layers.hpp,
or vision_layers.hpp. Include an inline implementation oftype and
the *Blobs() methods to specify blob number requirements. Omit the *_gpu declarations
if you'll only be implementing CPU code.
Implement your layer in layers/your_layer.cpp.
SetUp for initialization: reading parameters, allocating buffers, etc.
Forward_cpu for the function your layer computes
Backward_cpu for its gradient
(Optional) Implement the GPU versions Forward_gpu and Backward_gpu in layers/your_layer.cu.
Add your layer to proto/caffe.proto, updating the next available ID. Also declare
parameters, if needed, in this file.
Make your layer createable by adding it to layer_factory.cpp.
Write tests in test/test_your_layer.cpp. Use test/test_gradient_check_util.hpp to
check that your Forward and Backward implementations are in numerical agreement.
上面是一个大致的流程,我就直接翻译过来吧,因为我自己琢磨出来的步骤跟这个是一样的。在这
里,我们就添加一个Wtf_Layer,然后作用跟Convolution_Layer一模一样。注意这里的命名方式,Wtf第一个字母大写,剩下的小
写,算是一个命名规范吧,强迫症表示很舒服。
1. 首先确定要添加的layer的类型,是common_layer 还是
data_layer 还是loss_layer, neuron_layer, vision_layer
,这里的Wtf_Layer肯定是属vision_layer了,所以打开vision_layers.hpp
然后复制convolution_layer的相关代码,把类名还有构造函数的名字改为WtfLayer,如果没有用到GPU运算,那么把里面的带GPU
的函数都删掉
2. 将Wtf_layer.cpp 添加到src\caffe\layers文件夹中,代码内容复制convolution_layer.cpp 把对应的类名修改(可以搜一下conv关键字,然后改为Wtf)
3. 假如有gpu的代码就添加响应的Wtf_layer.cu (这里不添加了)
4. 修改proto/caffe.proto文件,找到LayerType,添加WTF,并更新ID(新的ID应该是34)。假如说Wtf_Layer有参数,比如Convolution肯定是有参数的,那么添加WtfParameter类
5. 在layer_factory.cpp中添加响应的代码,就是一堆if ... else的那片代码
6. 这个可以不做,但是为了结果还是做一个,就是写一个测试文件,检查前向后向传播的数据是否正确。gradient_check的原理可以参考UFLDL教程的对应章节
之后我会更新我自己写的maxout_layer的demo,在这立一个flag以鞭策自己完成吧╮(╯▽╰)╭
(二) 如何添加maxout_layer
表示被bengio的maxout给搞郁闷了,自己摆出一个公式巴拉巴拉说了一堆,结果用到卷积层的maxout却给的另一种方案,吐槽无力,不过后来又想了下应该是bengio没表述清楚的问题。
我的maxout的算法思路是这样的,首先要确定一个group_size变量,表示
最大值是在group_size这样一个规模的集合下挑选出来的,简而言之就是给定group_size个数,取最大。确定好group_size变量,
然后让卷积层的output_num变为原来的group_size倍,这样输出的featuremap的个数就变为原来的group_size倍,然后
以group_size为一组划分这些featuremap,每组里面挑出响应最大的点构成一个新的featuremap,这样就得到了maxout层的
输出。
Here's roughly the process I follow.
Add a class declaration for your layer to the appropriate one of common_layers.hpp, data_layers.hpp,loss_layers.hpp, neuron_layers.hpp,
or vision_layers.hpp. Include an inline implementation oftype and
the *Blobs() methods to specify blob number requirements. Omit the *_gpu declarations
if you'll only be implementing CPU code.
Implement your layer in layers/your_layer.cpp.
SetUp for initialization: reading parameters, allocating buffers, etc.
Forward_cpu for the function your layer computes
Backward_cpu for its gradient
(Optional) Implement the GPU versions Forward_gpu and Backward_gpu in layers/your_layer.cu.
Add your layer to proto/caffe.proto, updating the next available ID. Also declare
parameters, if needed, in this file.
Make your layer createable by adding it to layer_factory.cpp.
Write tests in test/test_your_layer.cpp. Use test/test_gradient_check_util.hpp to
check that your Forward and Backward implementations are in numerical agreement.
上面是一个大致的流程,我就直接翻译过来吧,因为我自己琢磨出来的步骤跟这个是一样的。在这
里,我们就添加一个Wtf_Layer,然后作用跟Convolution_Layer一模一样。注意这里的命名方式,Wtf第一个字母大写,剩下的小
写,算是一个命名规范吧,强迫症表示很舒服。
1. 首先确定要添加的layer的类型,是common_layer 还是
data_layer 还是loss_layer, neuron_layer, vision_layer
,这里的Wtf_Layer肯定是属vision_layer了,所以打开vision_layers.hpp
然后复制convolution_layer的相关代码,把类名还有构造函数的名字改为WtfLayer,如果没有用到GPU运算,那么把里面的带GPU
的函数都删掉
2. 将Wtf_layer.cpp 添加到src\caffe\layers文件夹中,代码内容复制convolution_layer.cpp 把对应的类名修改(可以搜一下conv关键字,然后改为Wtf)
3. 假如有gpu的代码就添加响应的Wtf_layer.cu (这里不添加了)
4. 修改proto/caffe.proto文件,找到LayerType,添加WTF,并更新ID(新的ID应该是34)。假如说Wtf_Layer有参数,比如Convolution肯定是有参数的,那么添加WtfParameter类
5. 在layer_factory.cpp中添加响应的代码,就是一堆if ... else的那片代码
6. 这个可以不做,但是为了结果还是做一个,就是写一个测试文件,检查前向后向传播的数据是否正确。gradient_check的原理可以参考UFLDL教程的对应章节
之后我会更新我自己写的maxout_layer的demo,在这立一个flag以鞭策自己完成吧╮(╯▽╰)╭
(二) 如何添加maxout_layer
表示被bengio的maxout给搞郁闷了,自己摆出一个公式巴拉巴拉说了一堆,结果用到卷积层的maxout却给的另一种方案,吐槽无力,不过后来又想了下应该是bengio没表述清楚的问题。
我的maxout的算法思路是这样的,首先要确定一个group_size变量,表示
最大值是在group_size这样一个规模的集合下挑选出来的,简而言之就是给定group_size个数,取最大。确定好group_size变量,
然后让卷积层的output_num变为原来的group_size倍,这样输出的featuremap的个数就变为原来的group_size倍,然后
以group_size为一组划分这些featuremap,每组里面挑出响应最大的点构成一个新的featuremap,这样就得到了maxout层的
输出。
推荐律师服务:
若未解决您的问题,请您详细描述您的问题,通过百度律临进行免费专业咨询